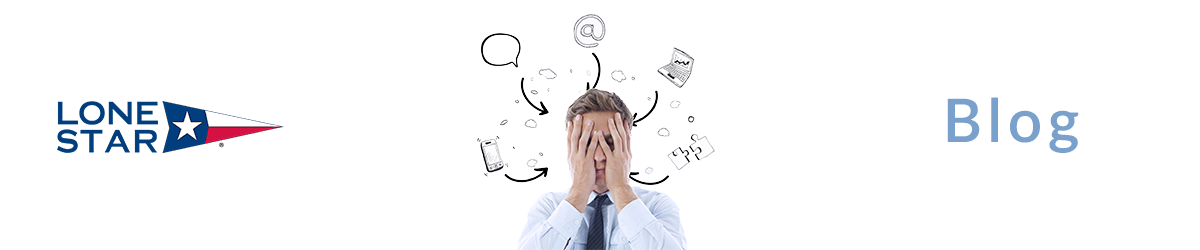
First Unsolved Problem in Data Science and Analytics
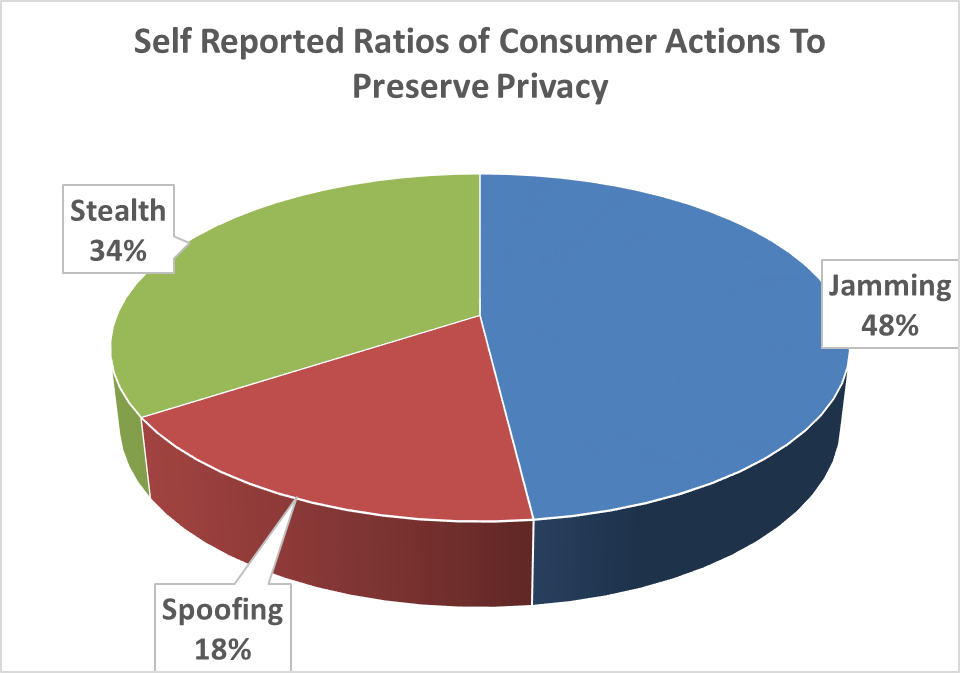
The first item on our list of seven unsolved problems is detecting dirty data.
It’s part of a larger problem; data quality. A Harvard Business Review article recently claimed only about 3% of corporate data meets basic quality standards.
Most studies suggest 80% of the time needed to solve a data science or analytics problem relates to finding and cleaning data. It’s the biggest hurdle we face. We probably can’t hope to get good at cleaning data unless we are good at finding dirt. So, let’s take a tour of a few dirty data types.
Some of it is falsified data generated automatically. The UK House of Lords thinks we need to prevent computer generated lies. Of course, that horse has been out of the barn for a long time. Weaponized bots on social media are powerful propaganda devices. At Lone Star, we studied this and blogged about it. Our nominal estimate is that state sponsored bots and trolls generate about 1.5 Trillion untruths per year. Of course, no one knows. But we think it seems likely there’s about 1 lie per person per day generated from a robot. Some of them are highly targeted.
Think of it; robots lying just for you.
This is one example of how hard it is to detect these lies. Facebook and Twitter have banned a few accounts. Our guess is these have already been replaced. They probably accounted for less than 10% of the problem because Russia is not the only nation who does this. More than a dozen nations do it, and the list is growing. It’s just a cheap way to spread your point of view, and promote both the truth and the lies that suit your national policy. It suits dictators especially well.
But it’s not just evil dictators who lie. We polled nearly 500 people. More than 80% of them said they took actions to protect privacy. We asked about eight specific actions, and on average, the people who did answer this question said they did about 3 of them.
We categorized their actions into three categories.
By the way, these are signal processing terms. That gives you a hint about how we think bad data might eventually be detected.
Stealth – about a third of the actions taken were in this category, which includes actions taken to avoid detection, like browsing incognito.
Jamming – about half the actions were in a category we called jamming. These actions try to break the tracking lock on a consumer. An example here is deleting cookies.
Spoofing – about one in 5 actions fall into this category. An example here is using a false name when filling out a form.
There are several fibs we didn’t ask about. A common fib is age. There are many others. But more importantly, people don’t tell the truth in polls. They lie more, drink more, smoke more and generally misbehave more than they will admit. It is nearly certain the problem is bigger than our data suggests.
So, intentional dirty data from “nice people” is an important category of dirty data, and, we have a hard time detecting it.
When you look at all these types of data dirt, it seems soil science knows more about dirt than data scientists. Soil scientists describe twelve recognized orders of soil in their taxonomy. These can be mapped into several sub-orders.
We don’t know if any taxonomy of different kinds of data dirt would help us perfectly identify dirty data. In fact, there are some good arguments, dating back to Babbage, this is not a perfectly solvable problem.
If someone can perfectly solve this problem, they deserve the equivalent of the Fields Medal in Math, or the Nobel for Physics.
But, more likely we don’t need to perfectly solve it. The GPS receiver in your car starts its work with a lot more noise than signal. Signal processing works well despite dirty signals.
But in signal processing, and in soil science, they have named their dirt. In data science, it’s an unsolved problem.
About Lone Star Analysis
Lone Star Analysis enables customers to make insightful decisions faster than their competitors. We are a predictive guide bridging the gap between data and action. Prescient insights support confident decisions for customers in Oil & Gas, Transportation & Logistics, Industrial Products & Services, Aerospace & Defense, and the Public Sector.
Lone Star delivers fast time to value supporting customers planning and on-going management needs. Utilizing our TruNavigator® software platform, Lone Star brings proven modeling tools and analysis that improve customers top line, by winning more business, and improve the bottom line, by quickly enabling operational efficiency, cost reduction, and performance improvement. Our trusted AnalyticsOSSM software solutions support our customers real-time predictive analytics needs when continuous operational performance optimization, cost minimization, safety improvement, and risk reduction are important.
Headquartered in Dallas, Texas, Lone Star is found on the web at http://www.Lone-Star.com.
Tags In
Recent Posts
- Lone Star Analysis Awarded Digital Twin Patent for Operations and Maintenance Applications
- The Steps to Laying Out an Embedded System Quantum Roadmap Featured in Military Embedded Systems
- Steve Roemerman Recognized as Aerospace AI Leader in Inaugural AI 75 List
- Lone Star Analysis Ltd. Sponsoring RAFA Rides 2024
- The Power of Predictive Modeling in Complex Problem Solving featured in COTS Journal