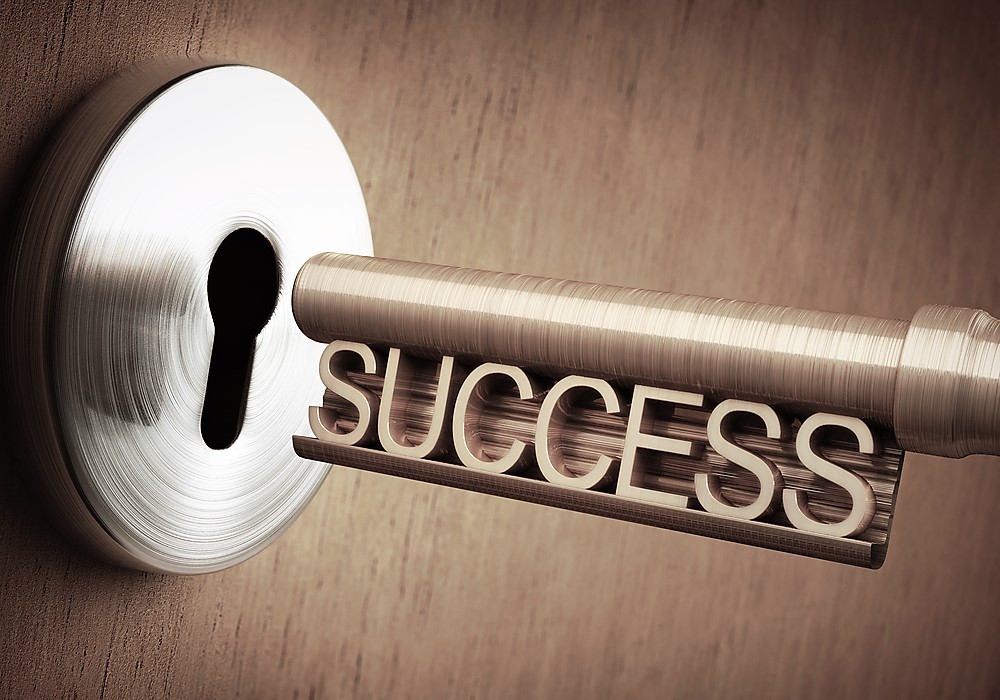
How Big Data Succeeds

How Big Data Succeeds
How Big Data Succeeds
Big Data analytics seems stuck. Spending grows, but returns on the spending are elusive. Gartner is among the most consistent chronicler of the challenge:
Big Data projects get stuck at the pilot stage; http://www.gartner.com/newsroom/id/3466117
Most projects will fail this year; http://www.gartner.com/newsroom/id/3130017
Of course, this prompts a great deal of writing. Topics range from “how to not fail” to, “how to take Big Data baby steps,” to “buy this stuff and your Big Data will magically succeed.”
The first thing that seems worth pointing out is the definition of “success” can vary.
The application of Artificial Intelligence to Big Data is a great example of defining success. As we’ve pointed out before, there are dozens of firms offering recommendation engines to help your customers shop on line. But they don’t work very well, and customers don’t like them. But Walmart and Amazon must use them. If they randomly recommend the millions of things they offer, they have a near zero chance of making a useful suggestion. So, being wrong even 90% of the time is a huge improvement.
You can read more about that, here – https://lone-star.com/artificial-intelligence-experiments-episode-4-using-ai-or-digital-twins/
And of course, AI using online unstructured data is prone to seduction by trolls, and errors; so, we can’t expect it to be really right all the time. Microsoft’s ill-fated Tay turned from being a chatty simulation of an adolescent, but quickly turned nasty when interacting with real humanity on Twitter. This idea is explored more fully here – https://lone-star.com/artificial-intelligence-experiments-episode-3-evil-ai/
But that may be alright if our goal is to be less wrong. Being less wrong is one way Big Data can succeed.
However, if we want to be mostly right, we need a more reliable cause-effect structure to our algorithm using our data feed.
That means we need some form of reliable provenance and ways to test the data stream for pollution. https://lone-star.com/where-did-this-come-from/ This may still employ some form of machine learning (call it AI if you like). But the established cause-effect relationships which G.E.P. Box called “mechanistic” must be preserved as foundational truth so our algorithm can’t become untethered like Tay did.
We think in many cases this means computing at the edge, in particular for IoT. And we mean real analytics at the edge, not just data packing. It is much harder to lose track of the data and its truth when we compute at the point of the data sources, and at the point of need. This is why Lone Star offers AnalyticsOSSM.
For longer term, big data, we think this usually mean a probabilistic digital twin.
Two projects we are working now will ingest the results from millions of transactions (in one case) and billions (in another), But this will be done in a highly-compressed form. Even that compressed form will be further reduced to a description of uncertainty (a probability distribution). We are delivering these with TruNavigator®.
Both TruNav™ and AnalyticsOSSM are designed for Boxian modeling – Cause and effect in the face of uncertainty. They are powerful ways Big Data can succeed.
About Lone Star Analysis
Lone Star Analysis enables customers to make insightful decisions faster than their competitors. We are a predictive guide bridging the gap between data and action. Prescient insights support confident decisions for customers in Oil & Gas, Transportation & Logistics, Industrial Products & Services, Aerospace & Defense, and the Public Sector.
Lone Star delivers fast time to value supporting customers planning and on-going management needs. Utilizing our TruNavigator® software platform, Lone Star brings proven modeling tools and analysis that improve customers top line, by winning more business, and improve the bottom line, by quickly enabling operational efficiency, cost reduction, and performance improvement. Our trusted AnalyticsOSSM software solutions support our customers real-time predictive analytics needs when continuous operational performance optimization, cost minimization, safety improvement, and risk reduction are important.
Headquartered in Dallas, Texas, Lone Star is found on the web at http://www.Lone-Star.com.
Recent Blog Posts
Tags In
Recent Posts
- Lone Star Analysis Awarded Digital Twin Patent for Operations and Maintenance Applications
- The Steps to Laying Out an Embedded System Quantum Roadmap Featured in Military Embedded Systems
- Steve Roemerman Recognized as Aerospace AI Leader in Inaugural AI 75 List
- Lone Star Analysis Ltd. Sponsoring RAFA Rides 2024
- The Power of Predictive Modeling in Complex Problem Solving featured in COTS Journal