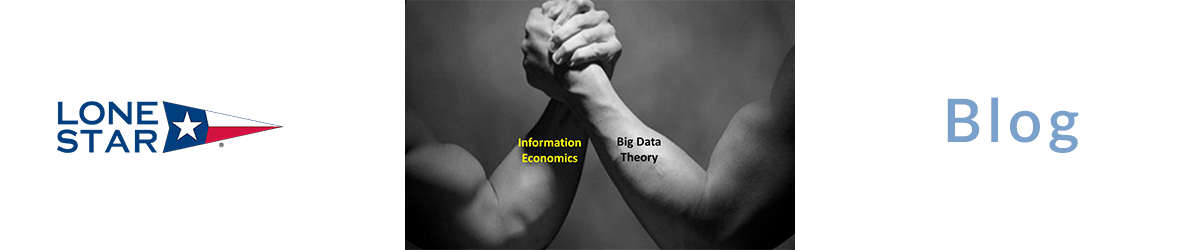
Information Economics Vs. Big Data
Two competing concepts are locked in a struggle. Most organizations who attempt digital transformation are engaged in this contest, even if they don’t know it.
What is this struggle? Information Economics is pitted against Big Data philosophy.
On one side we have Big Data theory. This holds with the idea “data is the new oil.” It’s the source of a great deal of Amazon’s value and nearly all of Facebook’s value. It’s very simple in some cases. When a data broker sells information about you, there is a value per record. Even Facebook, which is constantly changing can put a value on each relationship.
The data value can be very complicated. Amazon is constantly finding new ways to monetize its customer data. Innovative data use leads Big Data theorists to cling to masses of data that have no known purpose… yet. It leads to obsessive data hoarding.
On the other side, we have Information Economics. This concept has its own Wikipedia page which points out there are several subfields. Nearly all of them conflict with obsessive data hoarding. At some point, the costs of collecting and keeping hoarded data exceed its value.
An interesting version of information economics relates to a version of Decision Analysis. Popularized by Hubbard Decision Research, Applied Information Economics looks at both data costs, and the benefits of data use in analytics. Doug Hubbard is a persuasive spokesman, who points out the following:
- Most business data costs us something
- Most uses in analytics don’t require perfect knowledge
- Therefore, there is a point of diminishing returns in most data collection
A narrow way to think about this is that you learn the most from the first data you collect, even though you are left with some uncertainty. Each additional bit of data reduces uncertainty but gains less new knowledge. Amazon is often wrong when making a product recommendation, but that’s better than being wrong all the time, or never making recommendations. Many Big Data applications are working to achieve “being less wrong.”
A more general way to look at this is to think about costs and benefits. Industrial Internet of Things (IIoT) data, compared with human internet data provides an illustration.
Consumer internet and consumer IoT consolidate data while a profit can be generated. The data “cost” is zero, or even negative. This means the marginal cost to create new value streams is very low.
For IIoT, users self-fund everything. Information economics starts in a different place. The table doesn’t list all costs, of course. When we add the cost of data cleaning, curation, and provenance, costs can become very expensive.
So far, we’ve ignored the time value of money. It also matters a great deal. Consumer data and data collection is monetized very quickly. Industrial data is often much slower.
A recent example is the oil company that found even 1,000 wells of data didn’t provide enough Big Data to train a good production forecasting model using machine learning. The time to collect this data was long. Fortunately, most of it probably needed to be collected anyway.
Other IIoT applications are less forgiving. We’ve seen proposals to collect data for months, and even years with the ONLY purpose being to train a deep learning system. The economics of this are nearly always bad.
Lone Star serves several market verticals. We’ve seen the problem of flawed information economics play out in all of them. It’s one of the reasons we blogged last year on several components of IIoT and information economics; using Nyquist principles, thinking about data transport costs, and others.
We think there are many better alternatives for most IIoT applications than blind adherence to Big Data strategies born from the consumer, not industrial business models. IIoT has a wonderful promise. That promise is becoming reality for organizations who understand information economics.
About Lone Star Analysis
Lone Star Analysis enables customers to make insightful decisions faster than their competitors. We are a predictive guide bridging the gap between data and action. Prescient insights support confident decisions for customers in Oil & Gas, Transportation & Logistics, Industrial Products & Services, Aerospace & Defense, and the Public Sector.
Lone Star delivers fast time to value supporting customers planning and on-going management needs. Utilizing our TruNavigator® software platform, Lone Star brings proven modeling tools and analysis that improve customers top line, by winning more business, and improve the bottom line, by quickly enabling operational efficiency, cost reduction, and performance improvement. Our trusted AnalyticsOSSM software solutions support our customers real-time predictive analytics needs when continuous operational performance optimization, cost minimization, safety improvement, and risk reduction are important.
Headquartered in Dallas, Texas, Lone Star is found on the web at http://www.Lone-Star.com.
Tags In
Recent Posts
- Lone Star Analysis Awarded Digital Twin Patent for Operations and Maintenance Applications
- The Steps to Laying Out an Embedded System Quantum Roadmap Featured in Military Embedded Systems
- Steve Roemerman Recognized as Aerospace AI Leader in Inaugural AI 75 List
- Lone Star Analysis Ltd. Sponsoring RAFA Rides 2024
- The Power of Predictive Modeling in Complex Problem Solving featured in COTS Journal