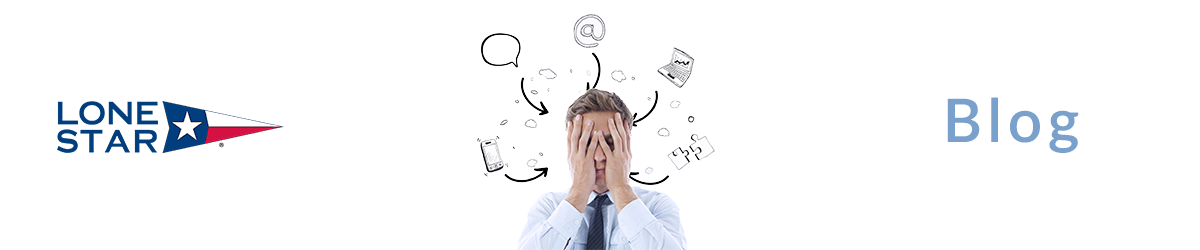
Seventh Unsolved Problem in Data Science and Analytics

Our 7th unsolved problem deals with the law.
Law is based on society’s norms and expectations.
We polled several hundred people and presented them with five hypothetical problems like this one. In each case, we described the algorithm as being “self-taught,” or being “based on strict rules”.
For the self-taught AI, we used the description you see here – “no one can fully explain how it works.”
What we saw was a clear bias against unexplainable AI. In this case, the jury is about 20% more inclined to rule against the car company. That’s worth hundreds of millions of dollars – maybe billions. Across all five areas we tested, the bias is even worse.
The spread grows to a 44% gap when we ask if it was fair that you didn’t get a loan, or an organ transplant based on an unexplainable algorithm.
People don’t like black boxes.
DARPA has recognized this with their efforts to make AI explainable. Lots of money is being spent to make AI explainable. So far, we don’t have a good solution for many of the most popular forms of AI (assuming we can even agree on what AI means).
On the other hand, some big firms have argued we should stop asking for AI to be explainable. Silly humans, you are holding up progress. But those same firms were among those whose results we could not reproduce. And new laws like the EU GDPR and likely new laws in California are going to create a challenge for unexplainable analytics, ML and AI.
To make matters worse, we found some other problems in our benchmarking. In the law, there are few good precedents to protect you from a plaintiff with a mathematically impossible premise. This is worse than just getting sued for a math non-sequitur. You can lose because a narrative fallacy seems so appealing, even if impossible. And, even if you win, it can take years to drag through courts.
One example relates to claims of discrimination.
If we have a robustly tested algorithm and use standard tests of confidence showing no discrimination by gender, zip code, eye color, etc., we are still not safe from claims of non-discrimination. If we divide a population into enough small groups, by age, gender, race and location, we’d expect that randomly some of them will SEEM to have been harmed.
And, this is just one example. There are other problems related to the lack of solid case law or statute precedent.
Perhaps a direr problem is the people creating algorithms and doing analytics. In our benchmarking we found most of them were unaware of their legal obligations. Worse they were guilty of breaching them.
It seems clear we are headed for serious legal problems in civil liability for uses like self-driving cars, and even criminal liability to protect individual rights and privacy.
Since we don’t have a clear set of semantics, and we don’t have a clear set of principles about what constitutes malpractice it’s easy to see this will end badly in some court cases. That is our 7th unsolved problem.
About Lone Star Analysis
Lone Star Analysis enables customers to make insightful decisions faster than their competitors. We are a predictive guide bridging the gap between data and action. Prescient insights support confident decisions for customers in Oil & Gas, Transportation & Logistics, Industrial Products & Services, Aerospace & Defense, and the Public Sector.
Lone Star delivers fast time to value supporting customers planning and on-going management needs. Utilizing our TruNavigator® software platform, Lone Star brings proven modeling tools and analysis that improve customers top line, by winning more business, and improve the bottom line, by quickly enabling operational efficiency, cost reduction, and performance improvement. Our trusted AnalyticsOSSM software solutions support our customers real-time predictive analytics needs when continuous operational performance optimization, cost minimization, safety improvement, and risk reduction are important.
Headquartered in Dallas, Texas, Lone Star is found on the web at http://www.Lone-Star.com.
Tags In
Recent Posts
- Lone Star Analysis Awarded Digital Twin Patent for Operations and Maintenance Applications
- The Steps to Laying Out an Embedded System Quantum Roadmap Featured in Military Embedded Systems
- Steve Roemerman Recognized as Aerospace AI Leader in Inaugural AI 75 List
- Lone Star Analysis Ltd. Sponsoring RAFA Rides 2024
- The Power of Predictive Modeling in Complex Problem Solving featured in COTS Journal