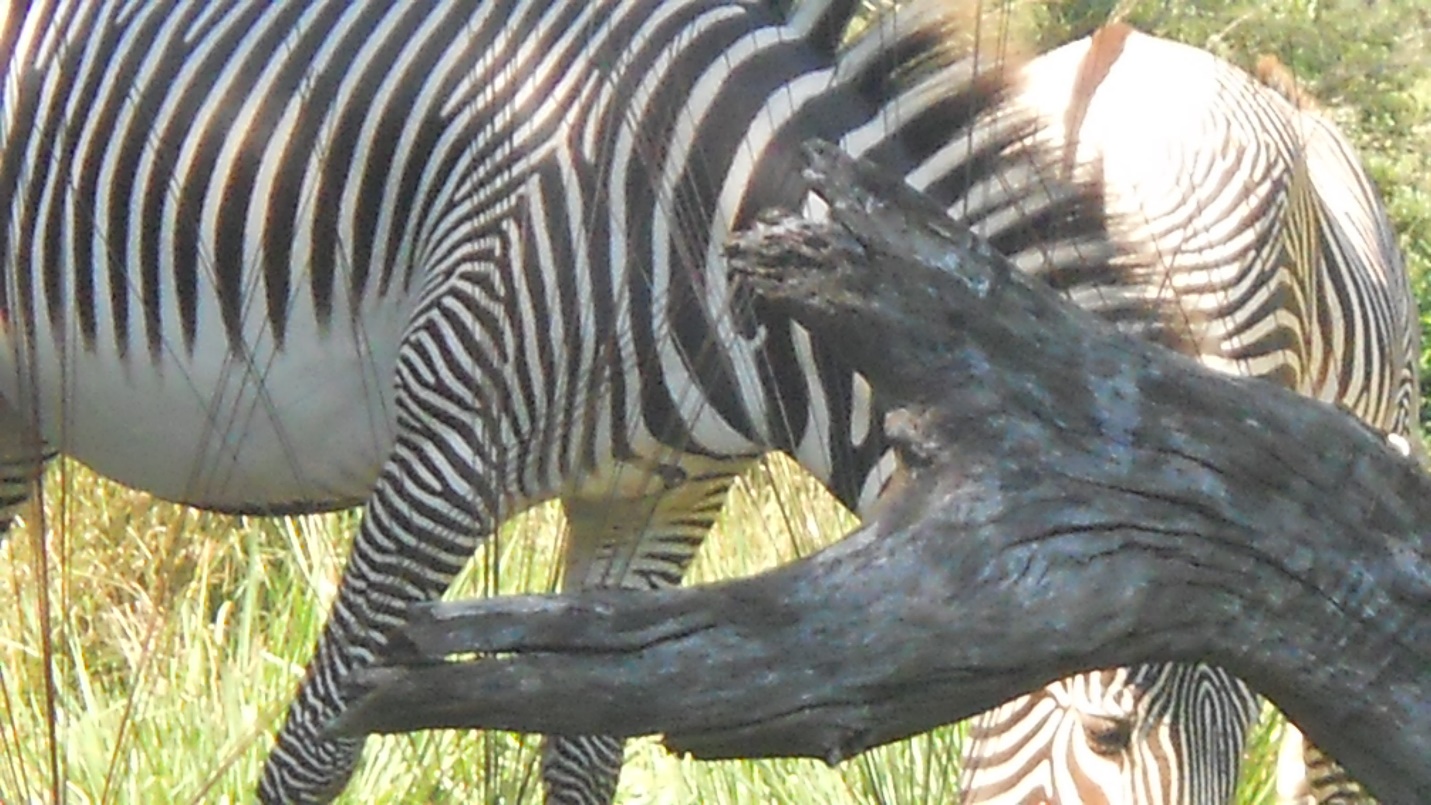
Artificial Intelligence Experiments – Episode #1; Errors and AI Prejudice

Artificial Intelligence Experiments – Episode #1; Errors and AI Prejudice
Artificial Intelligence Experiments – Episode #1; Errors and AI Prejudice
(Singularity Won’t Occur in 2017 – You know this is a zebra, right?)
Thanks to interest in trolling, fake news, and evil prejudice in AI, we thought it was time to share findings from ongoing experiments on Artificial Intelligence. This post deals with errors and prejudice. Come back later for more about fake news and trolling.
Recent test involved a well-known image search engine; https://images.google.com/ Google Images is really easy to use. It can search using an image as an input. It is fast, and hard to foul up. Since we wanted to involve several of our team, we wanted it to be easy.
Google Images has a great reputation. At a recent conference, a big data/AI evangelist suggested it was better than humans at classification. Not just a little better – the claim was Google could do a lot better.
Google’s “training set” of images is probably the largest sandbox for image algorithm training. Quora estimates Google has indexed about a trillion images; https://www.quora.com/How-many-pictures-has-Google-indexed. This is big data. A huge set of information, only roughly curated.
For our experiment, we submitted 46 images for classification.
The first batch was 27 pictures of ourselves. The results eased concern about an AI singularity taking over anytime soon. Of these 27 images, Google was just plain wrong 8 times; worse than 1 out of 3.
When we say “just plain wrong” we mean silly. Google identified our CEO as “Bunbury” an Australian city; https://en.wikipedia.org/wiki/Bunbury,_Western_Australia No doubt a wonderful place, but not Steve. Another co-worker submitted a picture decked out in a tuxedo. He was identified as “Queen Victoria” and “T-Shirt”. Another was labeled “obituary.” Other colleagues were identified as “YouTube” and “LinkedIn.”
More than half the time Google was “sort of right.” Humans chose a different description, but Google’s choice was understandable. One colleague submitted a picture wearing tinted eyeglasses. The result was “eyewear.” Google correctly identified only one person by name, and only three people were presented with pictures of themselves from the web.
Google also showed possible prejudice. All of the pictures of females came back with neutral or positive classifications. Professional headshots of women twice came were identified as “business executive.”
All negative connotations were ascribed to men. Some were in the label (“obituary” is hard to feel good about). Some were in the “similar images.” One executive was compared to the severed head of a pig. One tidy, tall man with close cropped hair was compared to a chubby fellow with several days of facial hair growth, moustache, and long greasy hair.
We wondered if, bowing to some early problems with supposed AI prejudice, Google added algorithm weighting for reverse prejudice? On the other hand, we would not quibble about our colleague whose image was classified as “beauty.” We agree with that (and her husband does too).
Images with no people were misclassified at a lower rate; only 2 of 19 were completely wrong, or about 11%. Remember, people pictures were completely wrong three times more frequently. Even with this small sample, it seems likely Google is better a “things” than “people.”
13 of the 19 images without people were “sort of right.” We tried to submit pictures which the AI evangelist claimed were Google’s strengths. For example, we submitted three pictures of birds. They were easily identifiable as storks, a duck, and pink flamingos. “Flamingo” was identified, but the others were just “bird” and, singular, even when more than one bird was present.
A strength, according to the AI evangelist was identifying equines (horses, donkeys zebras…). We felt zebras were least difficult, so we submitted three examples. They came back as “wildlife” or “terrestrial animal.” This was “sort of right” but three-year-old humans would say “zebra.” We tried a “real horse,” but Google was distracted by cattle in the background. We never saw a correct equine identification.
Supposedly we should have seen “zebra” or “horse” about 90% of the time. The odds our three zebra results were random bad luck, compared to the supposed odds are about a 1 in 1000 shot; the same odds given that Bono would become pope. That big data/AI booster was a tad optimistic.
Google seems to be doing pure image analysis. Performance didn’t change with file name or embedded text. This is a contrast; Facebook seems to use context, not just image processing. Either way, facial recognition helping television detectives (who load a grainy video surveillance image, and quickly get an ID), seems fictional.
In summary, AI might do better at classifying animal pictures than humans under controlled conditions. But “in the wild,” elementary school children do better. Also, our small sample size does not prove Google is sexist, but it is suggestive.
AI image classification will get better. But, classification is among the most promising topics for AI; we wonder how long it will take to mature in other areas. Other machine learning is more promising for Industrial Internet of Things (IIoT) applications where “Unexplainable is Unacceptable.”
Steve says he is not offended by being identified as that Aussie city.
G’Day mate?
About Lone Star Analysis
Lone Star Analysis enables customers to make insightful decisions faster than their competitors. We are a predictive guide bridging the gap between data and action. Prescient insights support confident decisions for customers in Oil & Gas, Transportation & Logistics, Industrial Products & Services, Aerospace & Defense, and the Public Sector.
Lone Star delivers fast time to value supporting customers planning and on-going management needs. Utilizing our TruNavigator® software platform, Lone Star brings proven modeling tools and analysis that improve customers top line, by winning more business, and improve the bottom line, by quickly enabling operational efficiency, cost reduction, and performance improvement. Our trusted AnalyticsOSSM software solutions support our customers’ real-time predictive analytics needs when continuous operational performance optimization, cost minimization, safety improvement, and risk reduction are important.
Headquartered in Dallas, Texas, Lone Star is found on the web at http://www.Lone-Star.com
Artificial Intelligence Experiments – Episode #1; Errors and AI Prejudice
Tags In
Recent Posts
- Lone Star Analysis Awarded Digital Twin Patent for Operations and Maintenance Applications
- The Steps to Laying Out an Embedded System Quantum Roadmap Featured in Military Embedded Systems
- Steve Roemerman Recognized as Aerospace AI Leader in Inaugural AI 75 List
- Lone Star Analysis Ltd. Sponsoring RAFA Rides 2024
- The Power of Predictive Modeling in Complex Problem Solving featured in COTS Journal